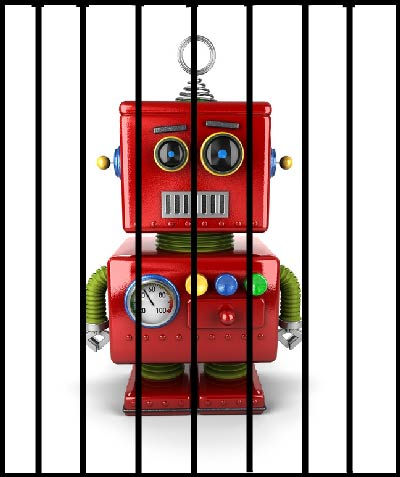
AI is popping up everywhere, and that’s not going to stop. Ever. And it only takes a few minutes in a news feed to find reasons to be nervous about this.
The dangers are many, and there is no shortage of voices articulating them:
AI will decimate the job market, destroying hundreds of millions of jobs and ending dozens of career options, crashing the global economy
AI will end up bursting out of containment and will seize control of the digital universe, then taking control of the actual universe
AI will blur the line between fake and real to the point that we can no longer tell the difference
AI will enable bad actors to irrevocably take control of our lives
AI will split society, even more than it already is, into a handful of ultra-rich elite Haves and a vast, powerless and miserable population of Have-Nots
That’s quite a list, and it isn’t even complete. It’s easy to get lost in any one of these and become deeply discouraged.
What can we do?
Unless and until AI takes control of our governments, we can do quite a bit.
Training Day
As it stands, there is one (and only one) core technology in the very broad domain of AI that has the power to change everything. It’s a technique called deep learning, which entails the building of complex digital models through mimicry of the human nervous system. This is the tech used to build the excellent vision systems and facial recognition applications, natural language processors, self-piloting vehicles, disease detection, drug synthesis algorithms, and other applications that are rapidly becoming ubiquitous.
The thing about deep learning systems, however, is that they rely upon vast amounts of data to be built at all – millions of sales transactions, millions of health records, millions of GPS points. The more data, the more accurate the resulting model; the less data, the weaker (and less useful) the model.
If new deep learning AI models can only be built with vast amounts of data – particularly public data – we can regulate the use of that data, making it legal to use it for AI model-building purposes only after scrutiny of its intended use, the methods to be employed, followed by a sign-off. With such a safeguard in place, fewer AI applications with the potential to do harm to the public will surface.
Taking Our Privacy Back
Along those lines, we are already generally concerned at how our personal data is harvested and exploited, in very cavalier fashion, by the Jeff Bezoses and Mark Zuckerbergs of the world. Many of us feel irritation, if not anger, when words we speak in the proximity of our smart phones trigger a flood of ads in our social media feeds. This invasiveness should make us angry; it is deeply Orwellian in practice, if not intent, for digital devices to spy on us without our permission.
We already need laws to curb this invasion and return power over our data to us, but if we can take that step, we will radically truncate the vast oceans of big data that AI builders are already leveraging to high heaven for their deep learning model-building. Sometimes that data is specific to us as individuals, as with the recommendation systems that eavesdrop on our preferences; but sometimes they drop our data into large buckets with others – buckets that would dry up, if personal data were forbidden to these AI builders.
And that’s before we get to bad actors. Reservoirs of personal data collected from millions of people enable the creation of propaganda-targeting systems that can be deployed in social media to swing elections. We know that this is already happening; we just don’t know to what extent.
Greatly strengthening privacy laws would put real controls on the data available for exploitation in AI models – and would be a major blow to those bad actors.
Deepfake ID
One of the most insidious applications of AI is deepfake, the creation of images and voices that are utterly convincing. This is a dangerous tool available to the bad actor, the propagandist who wants to flood social media with disinformation. A video of Donald Trump helping a nun cross a street, or a picture of Pete Buttigieg strangling a puppy could serve to alter perceptions within the electorate in a less-than-ideal manner.
We could, then, push for legislation that would require those who employ deepfake in any context – from music to television to social media – to identify it as such. Anyone who creates a deepfake and puts it out into the world needs to let the consumer know it’s deepfake.
A Fourth Asimovian Law
Isaac Asimov of I, Robot fame gave us the Three Laws of Robotics:
A robot may not injure a human being or, through inaction, allow a human being to come to harm.
A robot must obey the orders given it by human beings except where such orders would conflict with the First Law.
A robot must protect its own existence as long as such protection does not conflict with the First or Second Law.
Jonny Thomson, an Oxford philosophy professor writing for Big Think, proposes that Asimov missed one:
A robot must identify itself.
That is, when engaged with a human being, a robot must let the human being know it’s talking to a robot.
That’s a powerful idea. The line between people and chatbots is already hopelessly blurred, but at least we now assume the person in the chat isn’t actual. The day is upon us where we don’t know if the voice on the other end of the line is a human being or not. And since AI can now replicate human voices precisely, that’s another opportunity for bad actors to do incredible harm.
Let’s make the missing Asimov law a real law.
The argument can immediately be made that even if we enact all these laws here in the US, they certainly are not going to impose them in China or Russia. What good do the laws do if other countries don’t get on board?
Quite a bit, actually. If we have deepfake ID here in the US, then contradictory videos of actual events – real vs. deepfake – will be readily identified, and we’ll know that foreign actors are messing with us. If training data for deep learning models can’t be harvested from the actual target population, but has to be taken from, say, a foreign population, the resulting AI model won’t be nearly as accurate – making it all the more expensive for the model’s creator, to generate an inferior application.
These constraints are not, of course, absolute or fool-proof. They are stop-gap measures at best. But they’ll take us a long way, and keep us safer while we figure out something else.
Comments