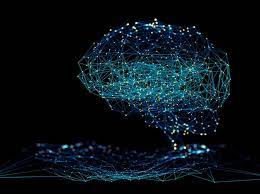
One aspect of the AI parade that I find both enjoyable and reassuring is the range of the response. While the world is tightly polarized, opinion-wise, by politics and economics and social issues, it is refreshingly all-over-the-place when it comes to the advent of artificial intelligence.
There are the chicken littles, terrified that AI will be the end of us. There are the opportunists, who see AI as a business multiplier that will supercharge the great capitalist machine. There are the just-another-revolution types, who see AI not as different-in-kind but simply the latest evolutionary bump in how we roll. And there are the tech snobs, phoo-phooing exemplars like ChatGPT as really nothing special and reminding everyone that all these shiny new toys are still light-years shy of the eventual inevitable singularity.
I love the variety, and am heartened that there are some domains where diversity of viewpoint prevails. But that’s not to say that I don’t find some viewpoints unsettling.
Two things about the AI disruption now underway really scare me, and one of them pings when dismissive pundits downplay the impact of AI. It’s a trigger thing, I freely admit, owing to my own long-term immersion in the subject and exacerbated by my peripheral studies of people brains. And it’s also a reflexive realization that all my life I’ve been witness to tech arriving today being ten times as good (and one-tenth as expensive) tomorrow.
The milestones used in most of these dismissives are usually AGI – artificial general intelligence – and deep learning. The former, we have yet to achieve. The latter is, of course, the AI breakthrough that changed the game: machine learning modeled on human neural networks, which became practical about a decade ago. Deep learning has given us visual systems that vastly outperform humans, facial recognition of uncanny sensitivity; natural language processing tremendously superior to what came before; and, in beta now, vehicle autonomy.
The dismissive here is that deep learning only serves to underscore the central argument that AI is no threat – like all AI, it can only do one thing well. As long as AI is a specialty technology that can’t move beyond its one-trick limitations, it can’t do much harm.
That brings up the other milestone, AGI. Artificial general intelligence will be AI that isn’t constrained to a single domain; it will be able to do a wide range of things, and do all of them better than humans. A single AGI will be able to assess a wide range of problems, across diverse domains, and generate better-than-human solutions to all of them. That’s something to be afraid of, certainly.
The dismissive response is that we’re still a long way from the advent of AGI - and, more specifically, that deep learning isn’t the AI tech that will lead to it. On this point, the experts side with the snobs.
Maciej Świechowski of the Systems Research Institute, Polish Academy of Sciences in Warsaw, for instance, makes the point that the neural models of today lack the logical mechanisms of yesterday’s AI – they can’t execute syllogistic reasoning. More than that, there is no pathway in deep learning tech to analogy, the this-is-like-that knowledge transfer mechanism that defines our own intelligence.
Data scientist Thuwaraskeh Murallie adds that even the most sophisticated AIs have no internal representation of the world to draw on, as humans and animals do. This is where analogies would be generated, if it were possible.
And futurist Tristan Greene points out that deep learning, in fact, runs the opposite direction of AGI: it requires oceans of data to build its solutions, while the entire point of AGI is that it can analyze and solve problems with a bare minimum of data.
None of this sets me at ease. It turns out, per Imran Ganaie et al of the University of Kashmir, that deep learning models can already be combined, a technique called ensemble learning, which takes its cues from ensemble modeling, a highly effective analytics technique for building predictive models. Deep learning models derived from this approach only require big data input for their initial modeling; the ensemble models require little data for learning thereafter.
Hungarian mathematician Tivadar Danka points out that neural networks are already training other neural networks, which opens the door to the next stage of deep learning evolution: layered models communicating with each other to solve problems, in much the same way that different regions of the human brain interact.
And DeepMind’s Gato, unveiled in May 2022, underscores the point that deep learning AIs aren’t confined to one task inherently; they are built that way because they are designed to do one thing well. Gato, a single model, can do more than 600 things well.
So, no, I’m not placated.
If deep learning AIs can learn from each other – and, moreover, pattern-map each other’s inner workings – then the abstraction that’s missing in Świechowski’s formulation can now emerge. If they can train each other and be combined into ensemble models, then the big data requirements go away – and they can, borrowing from one another, create larger and larger internal representations of their environment.
Inside deep learning AIs, there are layers of simulated neurons. The more layers, the more sensitive and granular the model. We can already build models exceeding our own neuron layers, and are within a few years of being able to exceed ourselves by orders of magnitude. So we can already build single-task models that outperform us wildly.
Imagine what we’ll have when those models are able to interact (which is already possible). To combine (which is already being done). To grow on their own. Which they’ll be able to do much sooner than most people realize.
There will be a quantum leap, where the models that outperform us wildly in isolated tasks will outperform us wildly as general AIs. Put another way, AGI won’t emerge in a gradual doggy-monkey-child-adult-superintelligence progression; it will emerge as superintelligence, right out of the box.
On top of it all, there are the experts sounding alarms who really know what they’re talking about, like Geoffrey Hinton, one of the pioneers of neural network design:
“We’ve entered completely unknown territory,” he recently said. “We’re capable of building machines that are stronger than ourselves, but we’re still in control. But what if we develop machines that are smarter than us? We have no experience dealing with these things.”
That’s starting to really scare me.
And there’s this other thing, too, but I’ll get into that later.
Comments